How to Interpret Sensitivity and Specificity
Sunday, June 19, 2022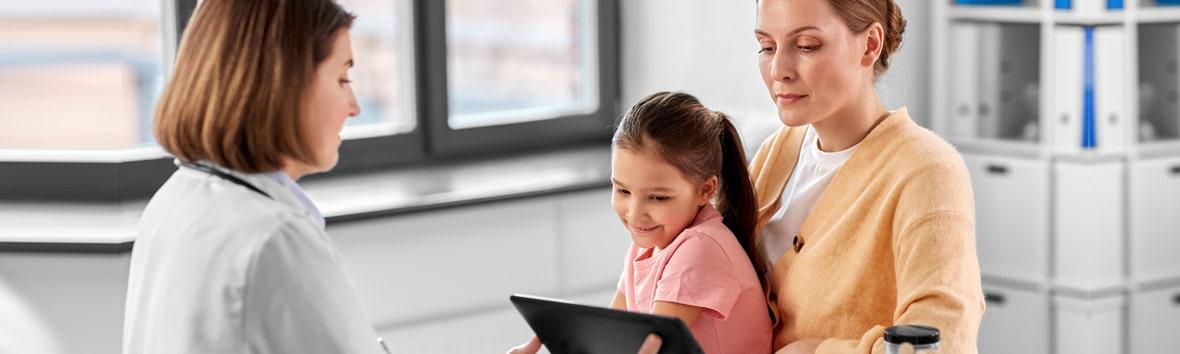
Assessments are an integral part of a helping professional’s work. The results obtained comprise one of the critical tools used in making high-stakes decisions, which can have a dramatic impact on the lives of those the helping professional serves. Thus, professionals rely on assessments to produce results that they can trust. There are many ways to measure whether a test is accurate or not. Most assessments include evaluations within the test manual describing multiple measures of validity and reliability. It is important for professionals to understand these results so that they can identify which tests to confidently add to their battery and for what purpose.
This paper begins with a review of reliability and validity before shifting focus to sensitivity and specificity as a measure of validity. The aim is to inform the reader as to what sensitivity and specificity measure, how they are determined, and what factors should be considered when evaluating these results in assessment manuals. Various characteristics of the sample can significantly impact the results of validity studies as well as what cut points are used when interpreting scores. The Comprehensive Assessment of Spoken Language, Second Edition (CASL-2) data were analyzed to demonstrate this. The results provide further support for the validity and utility of the CASL-2 as a diagnostic tool when measuring language abilities, while also remaining sensitive to those skills exhibited by individuals with mild symptoms of language impairment.
Reliability & Validity
Within the field of assessment, there are different types of reliability. Reliability refers to the consistency of a measure. If you gave the same test to the same person a few different times within a short period without changing any other features, would you get the same results? A common example of this is to think about a scale. If you step on a scale to measure your weight, and then you step on it again in 30 minutes, the numbers should be relatively the same. Obtaining multiple results that are corresponding (within an expected range) provides evidence that your scale is consistent, reliable. Another measure of accuracy is the validity of a test, which is the extent to which a test is measuring what it claims. Returning to the scale example, you just had your weight taken at the doctor’s office, where you know the measurement is accurate. Your bathroom scale gives the same number as the doctor’s scale. This indicates that your scale at home is valid.
A measure can be valid, in that it is measuring what you intended to measure, but not reliable. Your scale at home reads the same weight as your doctor’s office visit. After a few minutes you step on your scale again, but this time your weight is 10 pounds more than what the doctor’s scale said. Your scale is valid (it is still measuring weight), but it is not reliable (you did not gain 10 pounds in 10 minutes). Alternatively, a measure can be reliable, giving you consistent results every time, but not valid. Your scale at home is giving you the same result every time, but it is showing your weight is 10 pounds more than the doctor’s office scale said just a short while ago. This time, the scale is consistent (giving the same results each time), but it is not valid (the weight is not accurate).
Sensitivity & Specificity
Publishers include multiple measures of validity and reliability within the manual as part of the psychometric properties of the test. These are intended to help the reader understand the full scope of the test’s function and to support the use of the test. Professionals should review these studies prior to use to confirm that it is both reliable AND valid for their intended purpose. This is particularly important when using a test for diagnostic purposes.
Sensitivity and specificity are measures of validity. Sensitivity refers to a test’s ability to identify the presence of an actual deficit, condition, or disorder—a true positive result. Specificity refers to a test’s ability to identify the absence of an actual deficit, condition, or disorder—a true negative result. Figure 1 displays the possible test outcomes. Test results are considered “accurate” when the true positives and true negatives are both high, while the false positives and false negatives are both low. For a more advanced understanding of how sensitivity and specificity are calculated, sensitivity is the proportion of actual true positives identified [True Positives/(True Positives + False Negatives)]. Specificity is the proportion of actual true negatives identified [(True Negatives/(True Negatives + False Positives)].
The car alarm is a real-world example that is often used to illustrate this concept. In an ideal situation, your car alarm only sounds when someone is trying to break into your car, scaring them off and deterring a break in. This would be the top left cell of Figure 1, a True Positive occurrence. Conversely, you expect that your car alarm does not go off when there is no one breaking into your car. This is the bottom right cell of Figure 1, a True Negative. Though, we have all heard the incessant howl of a car alarm at 2am when there is no burglar present, but rather a cat jumped onto the roof of the car waking everyone except the owner of the vehicle. This is the top right cell, a False Positive. Perhaps worst of all, is when you are in earshot of your car alarm and know that it never sounded, yet you arrive at your parking space only to find that your car has been stolen. This is the bottom left cell, a False Negative.
Figure 1. Categories of Positive and Negative Test Results.
A perfect test would be one that is 100% sensitive (i.e., it identifies all people who actually have the condition of interest, top left cell) and 100% specific (it does not identify anyone who does not have the condition as having one, bottom right cell). However, most tests have some error rate (top right cell and bottom left cell), and there is usually a tradeoff between having a highly specific test and a highly sensitive one. Thinking again about the car alarm example, you don’t want it to go off every time a car drives by or a person walks near the car, or it would give you a lot of false positives. But you also don’t want the alarm to miss when someone attempts to steal the car and not go off, which would be a false negative. This is the tradeoff between sensitivity and specificity.
The same reasoning is true for assessment results. You want a test that correctly identifies someone who has a significant and meaningful skill deficit as having one (True Positive), and those who do not, should be identified as not having a deficit (True Negative). You also want the instances of a test incorrectly identifying someone as having a deficit when they do not (False Positive) and incorrectly identifying someone as typically performing when they actually do have a deficit (False Negative) to be as low as possible. High levels of both sensitivity and specificity indicate that the test is accurately identifying those who have the presence or absence of the condition of interest, while not mistakenly under- or over-identifying individuals.
Using Sensitivity & Specificity Statistics in Behavioral and Social Sciences
Although sensitivity and specificity are increasingly used to support the accuracy of behavioral and psychological assessments today, historically their use has been in medical and healthcare settings. This is particularly true for screening tests used to identify the likely presence or absence of a condition so that healthcare providers can make appropriate decisions for further testing and treatment (Trevethan, 2017). However, there are some fundamental differences to consider between medical conditions and psychological or behavioral conditions. These statistics were originally designed to detect the presence or absence of a condition, a yes or no to a diagnosis. For example, a Covid-19 test presents a result of positive or negative to indicate the presence or absence of the virus. Tests used in the behavioral and social sciences typically produce scores that exist along a range of values that have an order to them and often the distance between scores is meaningful, indicating changes in severity of symptoms. Many medical conditions have distinct categories: yes or no, you have a virus, or you do not. In contrast, many behavioral and psychological conditions typically exhibit a range of symptoms along a continuum or spectrum with more gray areas between the distinctive ends of yes or no, the condition is present or not.
While examining a variety of empirical studies has become an accepted framework for determining the validity of a diagnostic assessment (Dollaghan, 2004), Plante and Vance (1994) state that evaluating diagnostic accuracy should be the primary concern for a test used to identify language impairment. They refer to diagnostic accuracy as the test's ability to adequately identify those who have language impairments (the sensitivity) and identify those with typical language development (the specificity). To evaluate the sensitivity and specificity of behavioral and psychological assessments, a predetermined threshold must be used, often referred to as a “cutoff score” or “cut score” (Sheldrick et al., 2015). Again, this is because behavioral measures do not simply measure a condition with a yes or no category, but rather measure abilities or symptoms along a range. Often the cut scores are chosen in relation to the standard deviation (SD) of the test. For a test with a standard score mean of 100, 15 points in either direction is the standard deviation. Thus, a score of 85 would be 1SD below the mean and a score of 70 would be 2SDs below the mean.
Rather than presenting sensitivity and specificity values for a single cut score, Betz, Eickhoff, and Sullivan (2013) recommend providing results for multiple values so that professionals can choose a cutoff score best suited to their population. Often organizations differ in their criteria for determining the eligibility of services and when to continue or cease services. Presenting a range of values allows for flexibility and provides a different calibration for how sensitive you want your “alarm” to be set. You can customize it to fit your service population and the intended purpose of the test.
Interpreting Sensitivity & Specificity Statistics
Now that you know what sensitivity and specificity are, how can you evaluate these statistics in the tests you use? Every test that presents sensitivity and specificity statistics has conducted these analyses using a specific sample of interest, usually a clinical group, in comparison to another sample, usually a typically developing group. These samples matter and factors like age, diagnosis, and severity of deficits can have profound impacts on these statistics. It is important to review any demographics and descriptive data given about the samples used for the sensitivity and specificity analysis. These statistics are calculated using the instances of true positives and true negatives found in the samples. Thus, if the sample used in the analysis is not representative of the intended audience of the test, these statistics are meaningless in terms of supporting the validity of the test for the population intended.
Some characteristics to examine include demographics of the samples and the clinical features of the groups of interest. How many individuals were included in the study? Is the age range of those included in the analysis reflective of the entire age range of the test or were the analyses conducted on only a specific age group? How do the demographic characteristics of the samples included in the analysis compare to the overall test sample? How were the diagnoses determined for the clinical sample? What is the severity level of the cases included in the clinical sample?
To examine how these features can affect sensitivity and specificity, see Figure 2. A fictional test was administered to 10 individuals, age ranging from 5 to 21 years. Nine were previously diagnosed as having a speech-language impairment by 9 separate speech-language pathologists (SLPs), following the federal requirements for eligibility under the Individuals with Disabilities Education Act (IDEA) and their state regulations. The demographic characteristics of the sample roughly matched that of the recent US Census data, making it “representative” (although quite small). The SLPs made a clinical judgment as to the level of impairment being mild, moderate, or severe for each clinical case at the time of testing. Eight were rated as severely impaired and one as mildly impaired. There was one person identified as typically developing. Their standard scores on the test (M=100, SD=15) are given below.
Figure 2. Fictional Test, Sample of 10 Individuals.
Sensitivity and specificity differ depending on what value is used for the cutoff score. Using a cutoff of 2SDs below the mean, those who score above 70 would be considered typically developing while those who score at or below 70 are impaired. In this case, 8 out of the 9 individuals with diagnosed speech impairments were identified by this test. The sensitivity = 0.89 because 8 True Positives were correctly identified (score of 70 or below) divided by the 8 True Positives + 1 False Negative (mildly impaired case who was not identified). In other words, 89% of the sample was correctly identified as having a significant and meaningful language deficit. The one individual without a diagnosed speech-language impairment was correctly identified as not having a deficit in this example. The specificity is 1.00 because the one True Negative was correctly identified (score greater than 70) divided by the 1 True Negative + 0 False Positives. This means that 100% of the typically developing sample was correctly identified as not having a significant and meaningful language deficit.
What if the cutoff score was moved to a standard score of 80? The one mild case would then be identified, and the sensitivity and specificity of the test would both be 1.00. The less stringent cutoff of 80 provides improved sensitivity, capturing all those previously diagnosed as speech impaired. Also, it does not increase the chances of overidentifying those typically developing individuals (the specificity remains 1.00). The interpretive range for sensitivity and/or specificity states that .90–1.00 is good to excellent, while .80–.89 is considered acceptable for diagnostic measures (Plante & Vance, 1994). Thus, this fictional test would be perfect! But remember, the sample of this test is based on only 10 individuals. That is a tiny sample size and the majority (8 out of 10) have severe language impairments. How representative is this group to the population at large that you intend to test? Even though the numbers look excellent, you must consider the sample that is used in the data analysis to obtain these results.
Think about the referred individuals you see who do not already come to you with a diagnosis. If every possible case came through the door exhibiting severe deficits, a test would only confirm what you already can identify as a clinician. It is critical to examine the qualities of the sample used in analysis to determine if it is realistic and represents the diverse range of skills you might find in your population.
Sensitivity & Specificity in the CASL-2
The sensitivity and specificity analysis of the CASL-2 (Carrow-Woolfolk, 2017) is included alongside a variety of studies supporting the validity and reliability of the measure. The published analysis used the standardization sample compared to the clinical sample of 271 individuals, aged 3 to 21 years. Data collectors who participated in the standardization of the CASL-2 recruited individuals with the following disorders: expressive and/or receptive language disorder (n=72), hearing impairment (n=23), autism spectrum disorder (n=49), social (pragmatic) communication disorder (n=23), intellectual disability (n=36), learning disability (n=43), and developmental delay (n=25). To be included in the sample, these individuals needed to have a previously established clinical diagnosis (e.g., diagnosed by a professional according to federal and state regulations prior to participation in the study) and be receiving special services. Because of the inclusion criteria, the clinical sample was not expected to exactly replicate that of the U.S. Census demographic distribution. However, the sample does offer some diversity in terms of ethnicity and parental education level. Males outnumbered females, as is often the case in clinical samples. See Table 4.5 in the CASL-2 manual for the exact demographic composition of the clinical sample.
The CASL-2 sensitivity and specificity analysis (presented in Table 5.20 of the CASL-2 manual) is replicated here for reference as Table 1. The analysis demonstrates the ability of the CASL-2 to accurately identify those with a clinical diagnosis from those who do not by using the CASL-2 General Language Ability Index (GLAI) standard score. It is important to note that the entire clinical sample was included in this published analysis. The rationale for including all clinical groups was to display the range of sensitivity and specificity in a very diverse population with a wide range of symptoms and ability.
Table 1. Published CASL-2 Sensitivity and Specificity Values Using a Diverse Clinical Sample. |
||
SS cutoff |
Sensitivity |
Specificity |
70 |
.41 |
.99 |
75 |
.47 |
.96 |
80 |
.64 |
.91 |
85 |
.74 |
.84 |
90 |
.86 |
.76 |
Notice that the range of sensitivity values does not meet the acceptable mark of .80 or greater (Plante & Vance, 1994) until the cutoff of a standard score of 90, which would be a lenient cutoff for most. Indeed, at a cutoff of 90, the specificity drops to .76, meaning the risk for over-identifying those in the typically developing group increases. The sensitivity values are much lower at the most stringent cutoff of 70 and 75, which suggests that many of the mild- to moderately-impaired individuals will not be identified using the CASL-2 GLAI score within this diverse group of clinical cases used in this analysis. The cutoff of 85 (1SD below the mean), provides a better balance capturing 74% of the clinical sample (closer to the .80 recommendation) and an acceptable specificity rate where 84% of the typically developing sample is accurately identified. These values are representative of the most commonly used assessments of language, and this is likely due to the diverse nature of the clinical samples included in these studies.
To demonstrate the impact that the sample of interest has on sensitivity and specificity, we analyzed the CASL-2 clinical sample again, but this time trimming the clinical group based on the type of clinical classification and the severity of the symptoms. The data collectors who participated in the CASL-2 standardization study rated the severity of symptoms for each of the participants who were previously given a clinical diagnosis. They rated everyone from the clinical group as mild, moderate, or severe based on the testing session where the practicing SLPs administered all available CASL-2 tests for the examinee’s age. Although this rating is somewhat subjective, we can presume the clinical judgment of the SLPs is sufficient to determine a discrepancy between those rated as mild compared to severe. As such, Table 2 presents the sensitivity and specificity values when the CASL-2 GLAI scores are compared for the typically developing standardization sample to only those who displayed moderate to severe symptoms, across all clinical groups.
Table 2. CASL-2 Sensitivity and Specificity Values Using a Moderate to Severe Clinical Sample. |
||
SS cutoff |
Sensitivity |
Specificity |
70 |
.53 |
.99 |
75 |
.64 |
.99 |
80 |
.84 |
.98 |
85 |
.92 |
.92 |
90 |
.99 |
.82 |
Note. The analyzed sample included 195 clinically diagnosed individuals and 2,194 typically developing individuals. |
These results demonstrate that a cutoff score of as low as 80 would be acceptable, with a sensitivity of .84 and specificity of .98. This means that 84% of the clinical sample was correctly identified as having language deficits, even across a diverse clinical sample, while 16% were missed and not identified as belonging to the clinical group when they should have been. Almost all typically developing individuals (98%) were correctly identified as not having language deficits, and only 2% were identified as having a deficit when they actually did not because their score fell below the cutoff of 80.
Using a very stringent cutoff score of 70 would only capture 53% of the clinical group in this case. This is due to the variance of the moderately impaired clinical group, whose scores fell between 71 to 85. This is highlighted by the increasing sensitivity values going from a cutoff of 70 to a cutoff of 85. As the cut score increases, more and more of the clinical cases are correctly identified such that at a cut score of 85 (1SD below the mean) sensitivity increases to .92. This means that 92% of the clinical sample included in this analysis was accurately identified, while 92% of the typically developing group was correctly identified as not having a deficit. This suggests that using very stringent cut scores such as 1.5 to 2SDs below the mean increases the risk of false negatives, or under-identifying individuals with actual language impairments. There are nuances in symptomology and less pronounced impairments may be overlooked when only considering 2SDs below the mean as the criteria for diagnosis. Those with milder yet significant conditions may be more likely performing closer to the 1SD below the mean range.
All clinical classifications were included in the analysis above. However, we might not expect that certain groups, such as those with general learning disability or developmental delay, would show deficits specific to language. Although language difficulties may exist within their symptomology and related to their diagnosis, language deficits are not the focus of their condition. Thus, we again trimmed the moderate to severe clinical sample to only include those clinical conditions where language deficits are an expected and pronounced symptom (all groups except learning disability and developmental delay). These results are presented in Table 3.
Table 3. CASL-2 Sensitivity and Specificity Values Using a Moderate to Severe Language-Impaired Clinical Sample. |
||
SS cutoff |
Sensitivity |
Specificity |
70 |
.60 |
.99 |
75 |
.68 |
.99 |
80 |
.89 |
.98 |
85 |
.97 |
.92 |
90 |
1.00 |
.82 |
Note. The analyzed sample included 141 clinically diagnosed individuals and 2,194 typically developing individuals. |
These results demonstrate that a cutoff score of 80 would be very good for diagnostic accuracy, with a sensitivity of .89 and specificity of .98. This means that 89% of the clinical sample was correctly identified as having language deficits, with only 11% not being identified. Almost all typically developing individuals (98%) were correctly identified as not having language deficits, while only 2% were identified as having a deficit when they did not because their score fell below the cutoff of 80. Using the stringent cutoff of 70 would capture 60% of the clinical group in this case. Again, this is due to the variance of the moderately impaired clinical group who are scoring between 1SD and 2SDs below the mean. The cutoff of 85 (1SD below the mean) improves the sensitivity to .97, indicating that 97% of the clinical sample would be correctly identified whereas only 3% would be missed because they are scoring above 85. While sensitivity goes up using a cutoff of 85, specificity decreases somewhat to 92% of the typically developing group being correctly identified, while 8% would be identified as having a language impairment.
This tradeoff of sensitivity and specificity highlights how different cut points may be used in different situations. Using the cut scores of 80, 85, or 90 all provide acceptable choices for sensitivity and specificity. Depending on the intention of the test administration and the client who is being tested, one cut score may be more appropriate than another. For high-stakes eligibility cases, you may wish to use a more stringent cutoff at 80 (lower than 1SD below the mean) because approximately 89% of all individuals who exhibit moderate to severe language impairments will be accurately identified, while 98% of the typically developing individuals will be accurately identified. In contrast, when you are testing for treatment planning or lower-stakes decisions, you may wish to use a higher cut score, such as 85 or 90, that is much more inclusive of those who may experience more mild symptoms of language impairments but may also slightly increase the chances of overidentifying those in the typically developing population.
Summary
Measures of test validity are an important part of the overall examination of an assessment’s psychometric properties. To diagnose with confidence and accuracy, one must use a test that demonstrates reliability and validity across a range of empirical studies. One such measure of validity is the analysis of sensitivity and specificity. These statistics reflect the ability of a test to accurately identify those who truly have a deficit, condition, or disorder (sensitivity), while also correctly identifying those who do not (specificity). However, behavioral and psychological tests such as direct performance assessments often produce scores on a continuous scale where differences in score values are meaningful, compared to the discrete, nominal scores of many medical tests.
In order to calculate and use sensitivity and specificity values, cut points must be implemented as a threshold to determine the presence or absence of impairment. Generally, as you increase the value of the cutoff score you correctly identify more and more impaired individuals (higher sensitivity), but you also increase your chances of over-identification (lower specificity). Whereas, if you use very stringent cutoff scores you risk under-identifying impaired individuals (lower sensitivity), but you generally have higher specificity (you are not mistakenly identifying unimpaired individuals). This tradeoff between sensitivity and specificity is often a determinant for which cut point to use as a threshold in evaluating a test and for what purposes you intend to give the test results.
The samples used to calculate the sensitivity and specificity analysis can have a dramatic impact on the statistics. Professionals are encouraged to critically evaluate various descriptive features of the samples such as sample size, age, demographic characteristics, clinical diagnoses included, and severity of the clinical group being compared. A re-analysis of the CASL-2 clinical data was examined to illustrate this point. The CASL-2 sensitivity and specificity values were significantly improved when only those who exhibit more moderate to severe symptoms were included in the clinical sample. Sensitivity improved even more once only those clinical groups who might be expected to show deficits in language were included rather than the more general clinical sample. These results provide further support for the validity of the CASL-2 as a diagnostic measure of language impairment, while also supporting its use in identifying those who demonstrate more mild symptoms.
There is utility in using sensitivity and specificity for evaluating assessments of direct performance and behavior, particularly for identifying those at risk for negative outcomes based on ability levels or behavior. However, sensitivity and specificity should not be thought of as a precise measure of validity on its own. Further, the interpretation of these statistics is dependent entirely on what threshold is chosen as a cutoff (e.g., using 1, 1.5, or 2SDs below the mean). Studies have shown the chances of misclassifying individuals increases significantly as scores approach that decision threshold (Robins, 1985; Sheldrick et al., 2015; Spaulding, Plante, & Farinella, 2006; Swets, Dawes, & Monahan, 2000).
Professionals should take a variety of cut points into consideration, with cutoffs moving from more to less stringent depending on if the goal of the assessment is diagnostic to treatment planning. As with all assessments, a single test score should not be used in isolation for diagnosis or treatment planning. Instead, assessment results should be used in concert with other data (e.g., other assessment results, parent and teacher interviews, review of available records, direct observation, etc.) to identify a disorder or disability. The fuller the picture we can capture, the better able we are to illuminate the path forward for those individuals who are seeking our guidance as helping professionals.
Downloadable pdf version
Related Posts:
- The Benefits of Online Assessments, Scoring, and Intervention Monitoring
- How to Assess the Five Components of Reading This School Year
- How Do You Choose the Right Dyslexia Assessment for Your Student?
Research and Resources:
Betz, S. K., Eickhoff, J. R., & Sullivan, S. F. (2013). Factors influencing the selection of standardized tests for the diagnosis of specific language impairment. Language, Speech, and Hearing Services in Schools, 44, 133-146.
Carrow-Woolfolk, E. (2017). Comprehensive Assessment of Spoken Language, Second Edition (CASL-2). Torrance, CA: Western Psychological Services.
Dollaghan, C.A. (2004). Evidence-based practice in communication disorders: what do we know, and when do we know it? Journal of Communication Disorders, 37(5), 391-400.
Plante, E. & Vance, R. (1994). Selection of preschool language tests: A data-based approach. Language, Speech, and Hearing Services in Schools, 25, 15-24.
Robins, L.N. (1985). Epidemiology: reflections on testing the validity of psychiatric interviews. Archives of General Psychiatry, 42(9), 918–924.
Sheldrick, R. C., Benneyan, J. C., Giserman-Kiss, I., Briggs-Gowan, M. J., Copeland, W., and Carter, A. S. (2015). Thresholds and accuracy in screening tools for early detection of psychopathology. The Journal of Child Psychology & Psychiatry, 56(9), 936-948.
Spaulding, T. J., Plante, E., & Farinella, K. A. (2006). Eligibility criteria for language impairment: is the low end of normal always appropriate? Language, Speech, and Hearing Services in Schools, 37(1), 61-72.
Swets, J.A., Dawes, R.M., & Monahan, J. (2000). Psychological science can improve diagnostic decisions. Psychological Science in the Public Interest, 1(1), 1–26.
Trevethan, R. (2017). Sensitivity, specificity, and predictive values: foundations, liabilities, and pitfalls in research and practice. Frontiers in Public Health, November 20.